The recent surge in interest in generative AI highlights the critical role that AI will play in future wireless systems. With the transition to 5G, wireless systems have become increasingly complex and more challenging to manage, forcing the wireless industry to think beyond traditional rules-based design methods.
5G Advanced will expand the role of wireless AI across 5G networks introducing new, innovative AI applications that will enhance the design and operation of networks and devices over the next three to five years. Indeed, wireless AI is set to become a key pillar of 5G Advanced and will play a critical role in the end-to-end (E2E) design and optimization of wireless systems. In the case of 6G, wireless AI will become native and all-pervasive, operating autonomously between devices and networks and across all protocols and network layers.
E2E Systems Optimization
AI has already been used in smartphones and other devices for several years and is now increasingly being used in the network. However, AI is currently implemented independently, i.e. either on the device or in the network. As a result, E2E systems performance optimization across devices and network has not been fully realized yet. One of the reasons for this is that on-device AI training has not been possible until recently.
On-device AI will play a key role in improving the E2E optimization of 5G networks, bringing important benefits for operators and users, as well as overcoming key challenges. Firstly, on-device AI enables processing to be distributed over millions of devices thus harnessing the aggregated computational power of all these devices. Secondly, it enables AI model learning to be customized to a particular user’s personalized data. Finally, this personalized data stays local on the device and is not shared with the cloud. This improves reliability and alleviates data sovereignty concerns. On-device AI will not be limited to just smartphones but will be implemented across all kinds of devices from consumer devices to sensors and a plethora of industrial equipment.
New AI-native processors are being developed to implement on-device AI and other AI-based applications. A good example is Qualcomm’s new Snapdragon X75 5G modem-RF chip, which has a dedicated hardware tensor accelerator. Using Qualcomm’s own AI implementation, this Gen 2 AI processor boosts the X75’s AI performance more than 2.5 times compared to the previous Gen 1 design.
While on-device AI will play a key role in improving the E2E performance of 5G networks, overall systems optimization is limited when AI is implemented independently. To enable true E2E performance optimization, AI training and inference needs to be done on a systems-wide basis, i.e. collaboratively across both the network and the devices. Making this a reality in wireless system design requires not only AI know-how but also deep wireless domain knowledge. This so-called cross-node AI is a key focus of 5G Advanced with a number of use cases being defined in 3GPP’s Release 18 specification and further use cases expected to be added in later releases.
Wireless AI: 5G Advanced Release 18 Use Cases
3GPP’s Release 18 is the starting point for more extensive use of wireless AI expected in 6G. Three use cases have been prioritized for study in this release:
- Use of cross-node Machine Learning (ML) to dynamically adapt the Channel State Information (CSI) feedback mechanism between a base station and a device, thus enabling coordinated performance optimization between networks and devices.
- Use of ML to enable intelligent beam management at both the base station and device, thus improving usable network capacity and device battery life.
- Use of ML to enhance positioning accuracy of devices in both indoor and outdoor environments, including both direct and ML-assisted positioning.
Channel State Feedback:
CSI is used to determine the propagation characteristics of the communication link between a base station and a user device and describes how this propagation is affected by the local radio environment. Accurate CSI data is essential to provide reliable communications. With traditional model-based CSI, the user device compresses the downlink CSI data and feeds the compressed data back to the base station. Despite this compression, the signalling overhead can still be significant, particularly in the case of massive MIMO radios, reducing the device’s uplink capacity and adversely affecting its battery life.
An alternative approach is to use AI to track the various parameters of the communications link. In contrast to model-based CSI, a data driven air interface can dynamically learn from its environment to improve performance and efficiency. AI-based channel estimation thus overcomes many of the limitations of model-based CSI feedback techniques resulting in higher accuracy and hence an improved link performance. The is particularly effective at the edges of a cell.
Implementing ML-based CSI feedback, however, can be challenging in a system with multiple vendors. To overcome this, Qualcomm has developed a sequential training technique which avoids the need to share data across vendors. With this approach, the user device is firstly trained using its own data. Then, the same data is used to train the network. This eliminates the need to share proprietary, neural network models across vendors. Qualcomm has successfully demonstrated sequential training on massive MIMO radios at its 3.5GHz test network in San Diego (Exhibit 1).
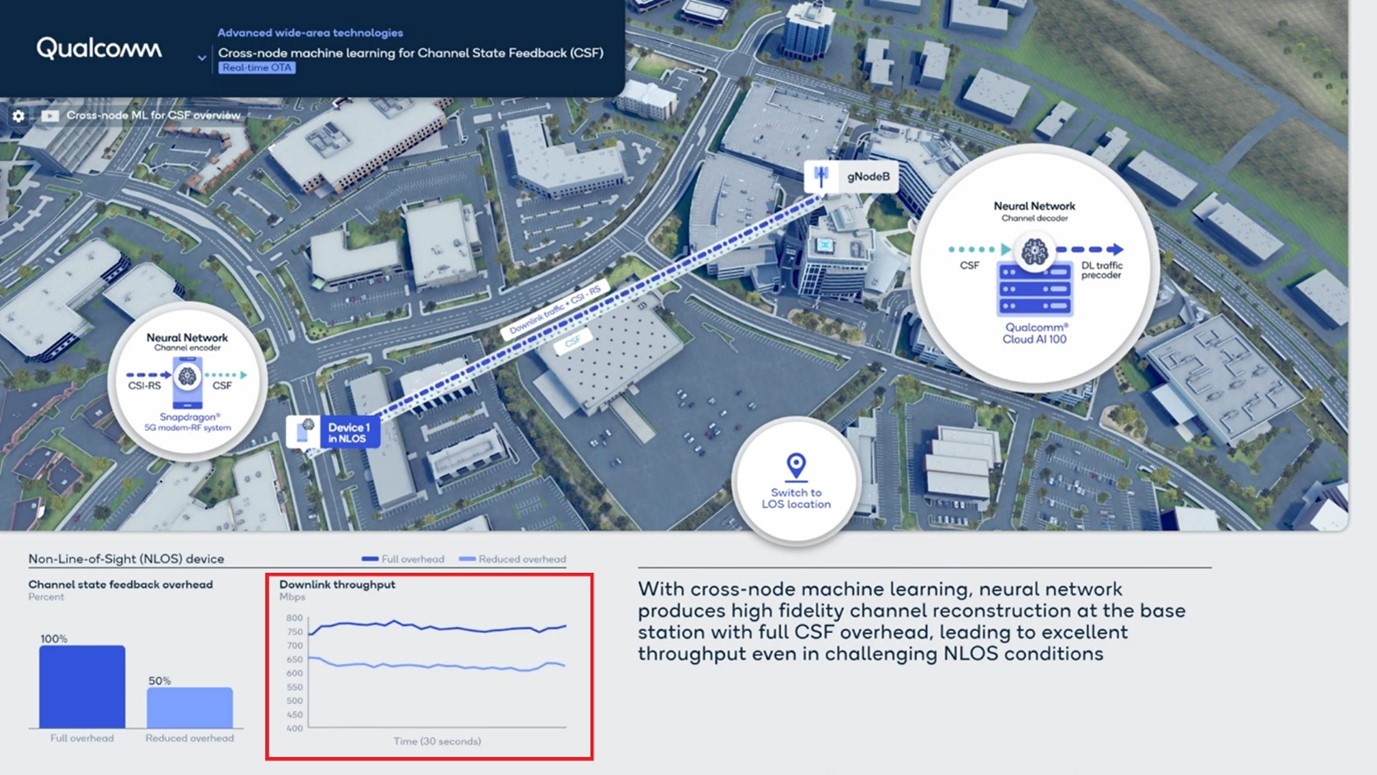
Exhibit 1: Realizing system capacity gain even in challenging non-LOS communication
AI-based Millimetre Wave Beam Management:
The second use case involves the use of ML to improve beam prediction on millimetre wave radios. Rather than continuously measuring all beams, ML is used to intelligently select the most appropriate beams to be measured – as and when needed. A ML algorithm is then used to predict future beams by interpolating between the beams selected – i.e. without the need to measure the beams all the time. This is done at both the device and the base station. As with CSI feedback, this improves network throughput and reduces power consumption.
Precise Positioning:
The third use case involves the use of ML to enable precise positioning. Qualcomm has demonstrated the use of multi-cell roundtrip (RTT) and angle-of-arrival (AoA)-based positioning in an outdoor network in San Diego. The vendor also demonstrated how ML-based positioning with RF finger printing can be used to overcome challenging non-line of sight channel conditions in indoor industrial private networks.
An AI-Native 6G Air Interface
6G will need to deliver a significant leap in performance and spectrum efficiency compared to 5G if it is to deliver even faster data rates and more capacity while enabling new 6G use cases. To do this, the 6G air interface will need to accommodate higher-order Giga MIMO radios capable of operating in the upper mid-band spectrum (7-16GHz), support wider bandwidths in new sub-THz 6G bands (100GHz+) as well as on existing 5G bands. In addition, 6G will need to accommodate a far broader range of devices and services plus support continuous innovation in air interface design.
To meet these requirements, the 6G air interface must be designed to be AI native from the outset, i.e. 6G will largely move away from the traditional, model-driven approach of designing communications networks and transition toward a data-driven design, in which ML is integrated across all protocols and layers with distributed learning and inference implemented across devices and networks.
This will be a truly disruptive change to the way communication systems have been designed in the past but will offer many benefits. For example, through self-learning, an AI-native air interface design will be able to support continuous performance improvements, where both sides of the air interface — the network and device — can dynamically adapt to their surroundings and optimize operations based on local conditions.
5G Advanced wireless AI/ML will be the foundation for much more AI innovation in 6G and will result in many new network capabilities. For instance, the ability of the 6G AI native air interface to refine existing communication protocols and learn new protocols coupled with the ability to offer E2E network optimization will result in wireless networks that can be dynamically customized to suit specific deployment scenarios, radio environments and use cases. This will a boon for operators, enabling them to automatically adapt their networks to target a range of applications, including various niche and vertical-specific markets.
Related Posts:
- Leveraging Technology To Create Greener Network
- 5G Advanced – Benefits and Opportunities for Operators
- 5G SA Core Tracker
- Global FWA & CPE Forecast by Technology and Regions
- Cloud RAN Platforms – Why Are Vendors Adopting Different Layer-1 Acceleration Strategies?
- Fixed Wireless Access and Consumer Premise Equipment Ecosystem and Forecast Update, April 2023
- Nvidia and Softbank Join Forces To Deploy AI-Based 5G MEC Telco Network Across Japan